Build Reactive REST APIs with Spring WebFlux – Part1
In this article, we will see how to build reactive REST APIs with Spring WebFlux. Before jumping into the reactive APIs, let us see how the systems evolved, what problems we see with the traditional REST implementations, and the demands from the modern APIs.
If you look at the expectations from legacy systems to modern systems described below,
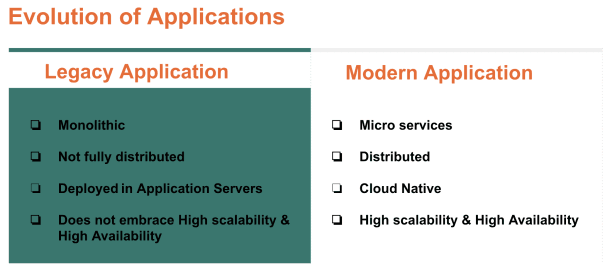
The expectations from the modern systems are, the applications should be distributed, Cloud Native, embracing for high availability, and scalability. So the efficient usage of system resources is essential. Before jumping into Why reactive programming to build REST APIs? Let us see how the traditional REST APIs request processing works.
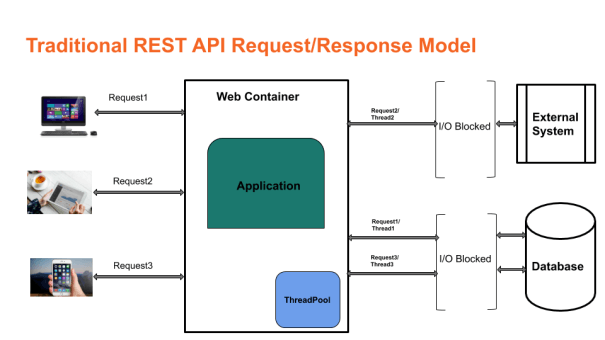
Below are the issues what we have with the traditional REST APIs,
- Blocking and Synchronous → The request is blocking and synchronous. The request thread will be waiting for any blocking I/O and the thread is not freed to return the response to the caller until the I/O wait is over.
- Thread per request → The web container uses thread per request model. This limits the number of concurrent requests to handle. Beyond certain requests, the container queues the requests that eventually impacts the performance of the APIs.
- Limitations to handle high concurrent users → As the web container uses thread per request model, we cannot handle high concurrent requests.
- No better utilization of system resources → The threads will be blocking for I/O and sitting idle. But, the web container cannot accept more requests. During this scenario, we are not able to utilize the system resources efficiently.
- No backpressure support → We cannot apply backpressure from the client or the server. If there is a sudden surge of requests the server or client outages may happen. After that, the application will not be accessible to the users. If we have backpressure support, the application should sustain during the heavy load rather than the unavailability.
Let us see how we can solve the above issues using reactive programming. Below are the advantages we will get with reactive APIs.
- Asynchronous and Non-Blocking → Reactive programming gives the flexibility to write asynchronous and Non-Blocking applications.
- Event/Message Driven → The system will generate events or messages for any activity. For example, the data coming from the database is treated as a stream of events.
- Support for backpressure → Gracefully we can handle the pressure from one system to on to the other system by applying backpressure to avoid denial of service.
- Predictable application response time → As the threads are asynchronous and non-blocking, the application response time is predictable under the load.
- Better utilization of system resources → As the threads are asynchronous and non-blocking, the threads will not be hogged for the I/O. With fewer threads, we could able to support more user requests.
- Scale based on the load
- Move away from thread per request → With the reactive APIs, we are moving away from thread per request model as the threads are asynchronous and non-blocking. Once the request is made, it creates an event with the server and the request thread will be released to handle other requests.
Now let us see how the Reactive Programming works. In the below example, once the application makes a call to get the data from a data source, the thread will be returned immediately and the data from the data source will come as a data/event stream. Here the application is a subscriber and the data source is a publisher. Upon the completion of the data stream, the onComplete event will be triggered.
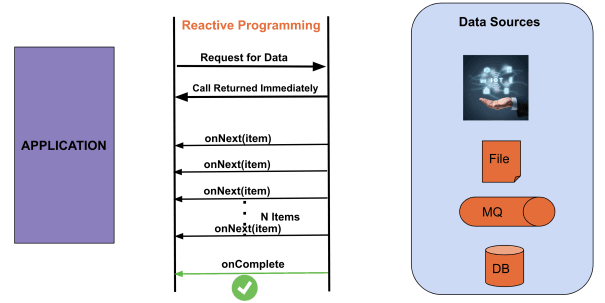
Below is another scenario where the publisher will trigger onError event if any exception happens.
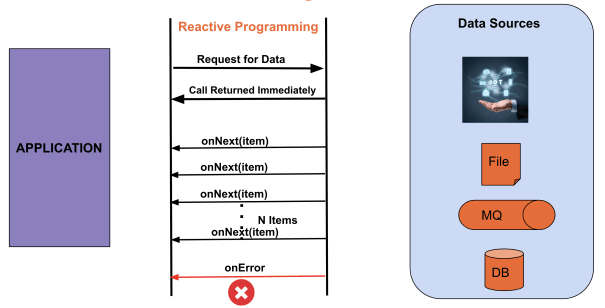
In some cases, there might not be any items to deliver from the publisher. For example, deleting an item from the database. In that case, the publisher will trigger the onComplete/onError event immediately without calling onNext event as there is no data to return.
Now, let us see what is backpressure? and how we can apply backpressure to the reactive streams? For example, we have a client application that is requesting data from another service. The service is able to publish the events at the rate of 1000TPS but the client application is able to process the events at the rate of 200TPS. In this case, the client application should buffer the rest of the data to process. Over the subsequent calls, the client application may buffer more data and eventually run out of memory. This causes the cascading effect on the other applications which depends on the client application. To avoid this the client application can ask the service to buffer the events at their end and push the events at the rate of the client application. This is called backpressure. The below diagram depicts the same.
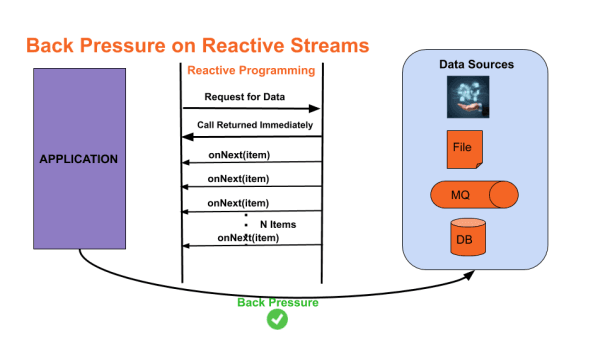
In the coming article, we will see the reactive streams specification and one of its implementation Project Reactor with some example applications. Till then, Happy Learning!!
Published on Java Code Geeks with permission by Siva Janapati, partner at our JCG program. See the original article here: Build Reactive REST APIs with Spring WebFlux – Part1 Opinions expressed by Java Code Geeks contributors are their own. |