Why Declarative Coding Makes You a Better Programmer
Declarative solutions with functional composition provide superior code metrics over legacy imperative code in many cases. Read this article and understand how to become a better programmer using declarative code with functional composition.
In this article, we will take a closer look at three problem examples and examine two different techniques (Imperative and Declarative) for solving each of these problems.
All source code in this article is open-source and available at
https://github.com/minborg/imperative-vs-declarative. In the end, we will also see how the learnings of this article can be applied in the field of database applications. We will use Speedment Stream as an ORM tool, since it provides standard Java Streams that correspond to tables, views and joins from databases and supports declarative constructs.
There is literally an infinite number of example candidates that can be used for code metrics evaluation.
Problem Examples
In this article, I have selected three common problems we developers might face over the course of our job days:
SumArray
Iterating over an array and perform a calculation
GroupingBy
Aggregating values in parallel
Rest
Implementing a REST interface with pagination
Solution Techniques
As implied at the beginning of this article, we will be solving said problems using these two coding techniques:
Imperative
An Imperative Solution in which we use traditional code styles with for-loops and explicitly mutable states.
Declarative
A Declarative Solution where we compose various functions to form a higher-order composite function that solves the problem, typically usingjava.util.stream.Stream
or variants thereof.
Code Metrics
The idea is then to use static code analysis applied to the different solutions using SonarQube (here SonarQube Community Edition, Version 7.7) ) so that we may derive useful and standardized code metrics for the problem/solution combinations. These metrics would then be compared.
In the article, we will be using the following code metrics:
LOC
“LOC” means “Lines-Of-Code” and is the number of non-empty lines in the code.
Statements
Is the total number of statements in the code. There could be zero to many statements on each code line.
Cyclomatic Complexity
Indicates the complexity of the code and is a quantitative measure of the number of linearly independent paths through a program’s source code. For example, a single “if” clause presents two separate paths through the code. Read more
here on Wikipedia.
Cognitive Complexity
SonarCube claims that “Cognitive Complexity breaks from the practice of using mathematical models to assess software maintainability. It starts from the precedents set by Cyclomatic Complexity, but uses human judgment to assess how structures should be counted and to decide what should be added to the model as a whole. As a result, it yields method complexity scores which strike programmers as fairer relative assessments of maintainability than have been available with previous models.” Read more here on SonarCube’s own page.
More often than not, it is desirable to conceive a solution where these metrics are small, rather than large.
For the record, it should be noted that any solution devised below is just one way of solving any given problem. Let me know if you know a better solution and feel free to submit a pull request via https://github.com/minborg/imperative-vs-declarative.
Iterating over an Array
We start off with an easy one. The object with this problem example is to compute the sum of the elements in an int array and return the result as along
. The following interface defines the problem:
1 2 3 4 | public interface SumArray { long sum( int [] arr); } |
Imperative Solution
The following solution implements the SumArray
problem using an imperative technique:
01 02 03 04 05 06 07 08 09 10 11 12 | public class SumArrayImperative implements SumArray { @Override public long sum( int [] arr) { long sum = 0 ; for ( int i : arr) { sum += i; } return sum; } } |
Declarative Solution
Here is a solution that implements SumArray
using a declarative technique:
1 2 3 4 5 6 7 8 9 | public class SumArrayDeclarative implements SumArray { @Override public long sum( int [] arr) { return IntStream.of(arr) .mapToLong(i -> i) .sum(); } } |
Note that IntStream::sum
only returns an int and therefore we have to apply the intermediate operation mapToLong()
.
Analysis
SonarQube provides the following analysis:
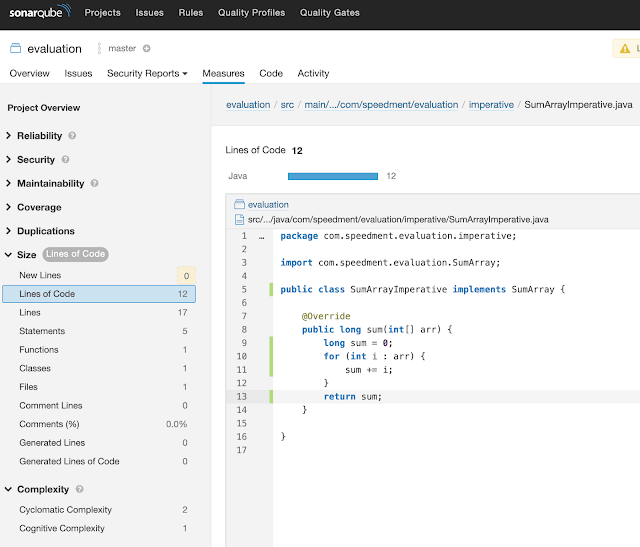
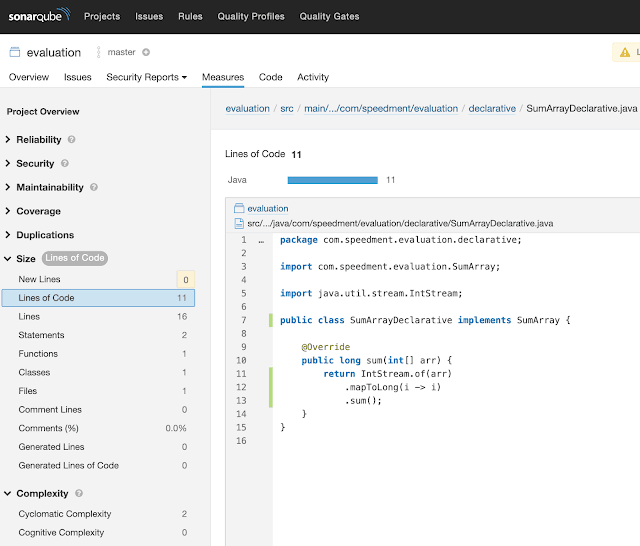
The code metrics for SumArray
are shown in the following table (lower is generally better):
Technique | LOC | Statements | Cyclomatic Complexity | Cognitive Complexity |
---|---|---|---|---|
Imperative | 12 | 5 | 2 | 1 |
Functional | 11 | 2 | 2 | 0 |
This is how it looks in a graph (lower is generally better):
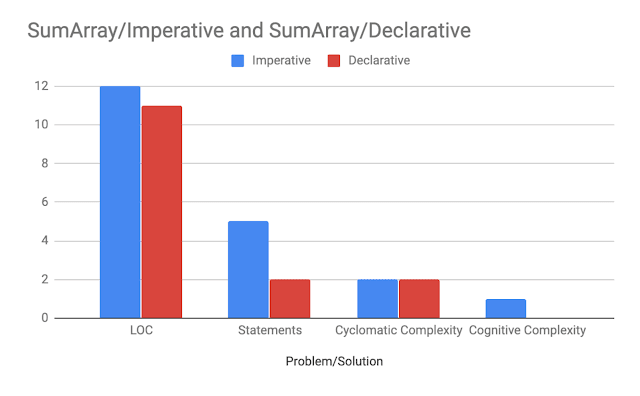
Aggregating Values in Parallel
The object with this problem example is to group Person
objects into different buckets, where each bucket constitutes a unique combination of the birth year of a person and the country that a person is working in. For each group, the average salary shall be computed. The aggregation shall be computed in parallel using the common ForkJoin pool.
This is how the (immutable) Person
class looks like:
01 02 03 04 05 06 07 08 09 10 11 12 13 14 15 16 17 18 19 20 21 22 23 24 25 26 27 28 | public final class Person { private final String firstName; private final String lastName; private final int birthYear; private final String country; private final double salary; public Person(String firstName, String lastName, int birthYear, String country, double salary) { this .firstName = requireNonNull(firstName); this .lastName = requireNonNull(lastName); this .birthYear = birthYear; this .country = requireNonNull(country); this .salary = salary; } public String firstName() { return firstName; } public String lastName() { return lastName; } public int birthYear() { return birthYear; } public String country() { return country; } public double salary() { return salary; } // equals, hashCode and toString not shown for brevity } |
We have also defined another immutable class called YearCountry
that shall be used as the grouping key:
01 02 03 04 05 06 07 08 09 10 11 12 13 14 15 | public final class YearCountry { private final int birthYear; private final String country; public YearCountry(Person person) { this .birthYear = person.birthYear(); this .country = person.country(); } public int birthYear() { return birthYear; } public String country() { return country; } // equals, hashCode and toString not shown for brevity } |
Having defined these two classes, we can now define this problem example by means of this interface:
1 2 3 4 5 | public interface GroupingBy { Map<YearCountry, Double> average(Collection<Person> persons); } |
Imperative Solution
It is non-trivial to implement an imperative solution to the GroupingBy
example problem. Here is one solution that solves the problem:
01 02 03 04 05 06 07 08 09 10 11 12 13 14 15 16 17 18 19 20 21 22 23 24 25 26 27 28 29 30 31 32 33 34 35 36 37 38 39 40 41 42 43 44 45 46 47 48 49 50 51 52 53 54 55 56 57 58 59 | public class GroupingByImperative implements GroupingBy { @Override public Map<YearCountry, Double> average(Collection<Person> persons) { final List<Person> personList = new ArrayList<>(persons); final int threads = ForkJoinPool.commonPool().getParallelism(); final int step = personList.size() / threads; // Divide the work into smaller work items final List<List<Person>> subLists = new ArrayList<>(); for ( int i = 0 ; i < threads - 1 ; i++) { subLists.add(personList.subList(i * step, (i + 1 ) * step)); } subLists.add(personList.subList((threads - 1 ) * step, personList.size())); final ConcurrentMap<YearCountry, AverageAccumulator> accumulators = new ConcurrentHashMap<>(); // Submit the work items to the common ForkJoinPool final List<CompletableFuture<Void>> futures = new ArrayList<>(); for ( int i = 0 ; i < threads; i++) { final List<Person> subList = subLists.get(i); futures.add(CompletableFuture.runAsync(() -> average(subList, accumulators))); } // Wait for completion for ( int i = 0 ; i < threads; i++) { futures.get(i).join(); } // Construct the result final Map<YearCountry, Double> result = new HashMap<>(); accumulators.forEach((k, v) -> result.put(k, v.average())); return result; } private void average(List<Person> subList, ConcurrentMap<YearCountry, AverageAccumulator> accumulators) { for (Person person : subList) { final YearCountry bc = new YearCountry(person); accumulators.computeIfAbsent(bc, unused -> new AverageAccumulator()) .add(person.salary()); } } private final class AverageAccumulator { int count; double sum; synchronized void add( double term) { count++; sum += term; } double average() { return sum / count; } } } |
Declarative Solution
Here is a solution that implements GroupingBy
using a declarative construct:
01 02 03 04 05 06 07 08 09 10 | public class GroupingByDeclarative implements GroupingBy { @Override public Map<YearCountry, Double> average(Collection<Person> persons) { return persons.parallelStream() .collect( groupingBy(YearCountry:: new , averagingDouble(Person::salary)) ); } } |
In the code above, I have used some static imports from theCollectors
class (e.g. Collectors::groupingBy
). This does not affect the code metrics.
Analysis
SonarQube provides the following analysis:
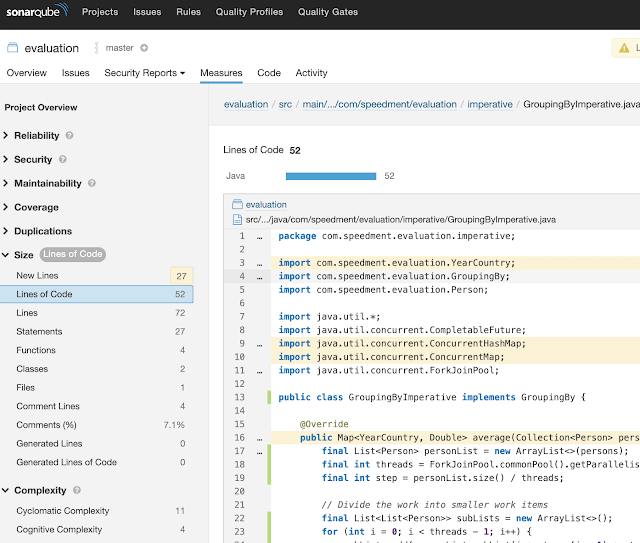
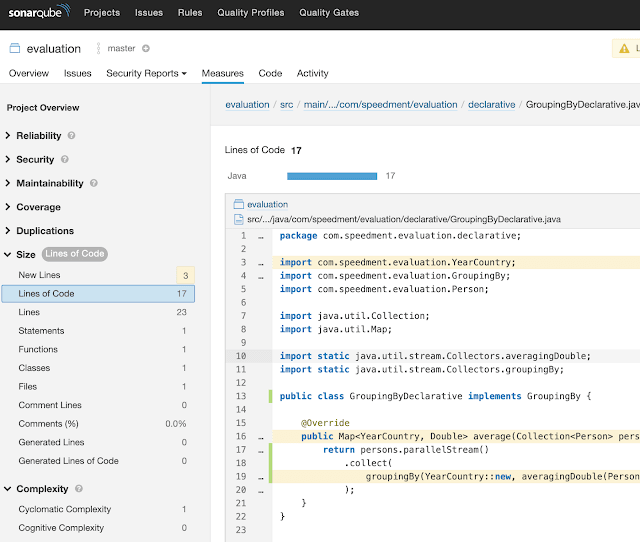
The code metrics for GroupingBy
are shown in the following table (lower is better):
Technique | LOC | Statements | Cyclomatic Complexity | Cognitive Complexity |
---|---|---|---|---|
Imperative | 52 | 27 | 11 | 4 |
Functional | 17 | 1 | 1 | 0 |
The corresponding graph looks like this (lower is generally better):
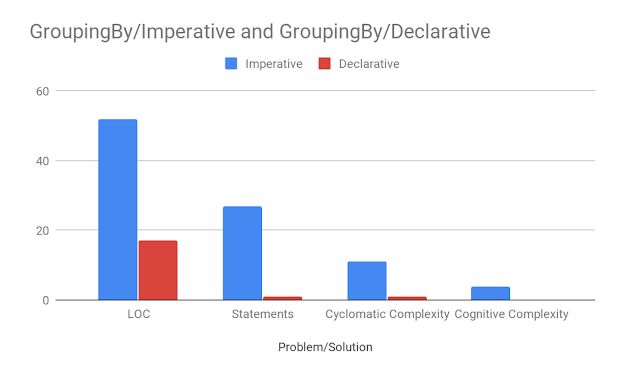
Implementing a REST Interface
In this exemplary problem, we are to provide a pagination service for Person objects. Persons appearing on a page must satisfy some (arbitrary) conditions and are to be sorted in a certain given order. The page shall be returned as an unmodifiable List of Person objects.
Here is an interface that captures the problem:
01 02 03 04 05 06 07 08 09 10 11 12 13 14 15 16 | public interface Rest { /** * Returns an unmodifiable list from the given parameters. * * @param persons as the raw input list * @param predicate to select which elements to include * @param order in which to present persons * @param page to show. 0 is the first page * @return an unmodifiable list from the given parameters */ List<Person> page(List<Person> persons, Predicate<Person> predicate, Comparator<Person> order, int page); } |
The size of a page is given in a separate utility class called RestUtil
:
1 2 3 4 5 | public final class RestUtil { private RestUtil() {} public static final int PAGE_SIZE = 50 ; } |
Imperative Solution
Here is an imperative implementation of the Rest interface:
01 02 03 04 05 06 07 08 09 10 11 12 13 14 15 16 17 18 19 20 21 | public final class RestImperative implements Rest { @Override public List<Person> page(List<Person> persons, Predicate<Person> predicate, Comparator<Person> order, int page) { final List<Person> list = new ArrayList<>(); for (Person person:persons) { if (predicate.test(person)) { list.add(person); } } list.sort(order); final int from = RestUtil.PAGE_SIZE * page; if (list.size() <= from) { return Collections.emptyList(); } return unmodifiableList(list.subList(from, Math.min(list.size(), from + RestUtil.PAGE_SIZE))); } } |
Declarative Solution
The following class implements the Rest interface in a declarative way:
01 02 03 04 05 06 07 08 09 10 11 12 13 14 15 | public final class RestDeclarative implements Rest { @Override public List<Person> page(List<Person> persons, Predicate<Person> predicate, Comparator<Person> order, int page) { return persons.stream() .filter(predicate) .sorted(order) .skip(RestUtil.PAGE_SIZE * ( long ) page) .limit(RestUtil.PAGE_SIZE) .collect(collectingAndThen(toList(), Collections::unmodifiableList)); } } |
Analysis
SonarQube provides the following analysis:
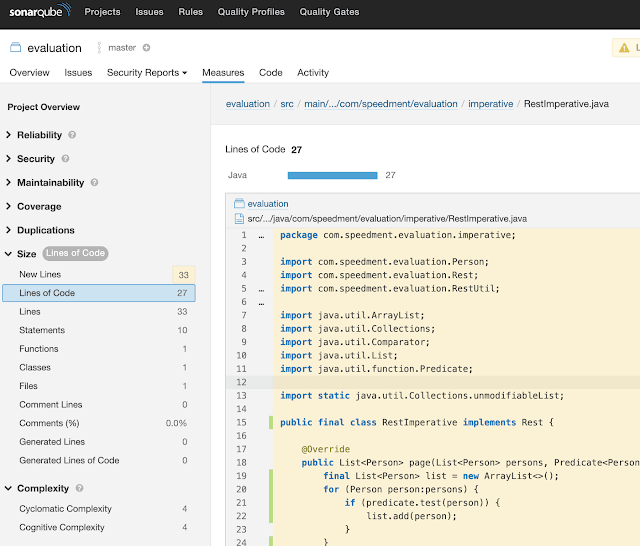
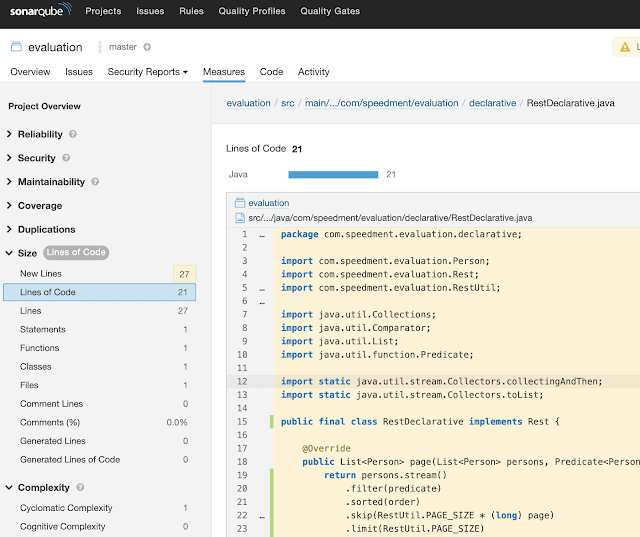
The following table shows the code metrics for Rest (lower is generally better):
Technique | LOC | Statements | Cyclomatic Complexity | Cognitive Complexity |
---|---|---|---|---|
Imperative | 27 | 10 | 4 | 4 |
Functional | 21 | 1 | 1 | 0 |
Here, the same numbers are shown in a graph (again lower is generally better):
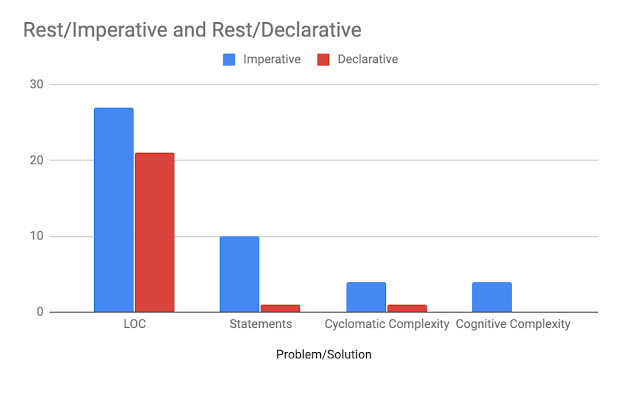
Java 11 Improvements
The examples above were written in Java 8. With Java 11, we could shorten our declarative code using LVTI (Local Variable Type Inference). This would make our code a bit shorter but would not affect code metrics.
1 2 3 4 5 6 7 | @Override public List<Person> page(List<Person> persons, Predicate<Person> predicate, Comparator<Person> order, int page) { final var list = new ArrayList<Person>(); ... |
Compared to Java 8, Java 11 contains some new collectors. For example, theCollectors.toUnmodifiableList()
which would make our declarative Rest solution a bit shorter:
01 02 03 04 05 06 07 08 09 10 11 12 13 14 | public final class RestDeclarative implements Rest { @Override public List<Person> page(List<Person> persons, Predicate<Person> predicate, Comparator<Person> order, int page) { return persons.stream() .filter(predicate) .sorted(order) .skip(RestUtil.PAGE_SIZE * ( long ) page) .limit(RestUtil.PAGE_SIZE) .collect(toUnmodifiableList()); } |
Again, this will not impact the code metrics.
Summary
Averaging the code metrics for our three exemplary problems yields the following result (lower is generally better) :
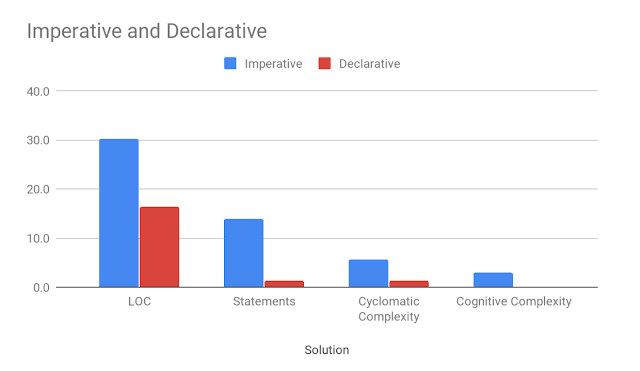
Given the input requirements in this article, there is a remarkable improvement for all code metrics when we go from imperative to declarative constructs.
Use Declarative Constructs in Database Applications
In order to reap the benefits of declarative constructs in database applications, we have used Speedment Stream. Speedment Stream is a Stream-based Java ORM tool that can turn any database table/view/join into Java streams and thereby allows you to apply your declarative skills in database applications.
Your database applications code will get much better. In fact, a pagination REST solution with Speedment and Spring Boot against a database might be expressed like this:
1 2 3 4 5 6 7 8 9 | public Stream<Person> page(Predicate<Person> predicate, Comparator<Person> order, int page) { return persons.stream() .filter(predicate) .sorted(order) .skip(RestUtil.PAGE_SIZE * ( long ) page) .limit(RestUtil.PAGE_SIZE); } |
Where the Manager<Person> persons
is provided by Speedment and constitutes a handle to the database table “Person” and can be @AutoWired
via Spring.
Conclusions
Choosing declarative over imperative solutions can reduce general code complexity massively and can provide many benefits including faster coding, better code quality, improved readability, less testing, reduced maintenance costs and more.
In order to benefit from declarative constructs within database applications, Speedment Stream is a tool that can provide standard Java Streams directly from the database.
Mastering declarative constructs and functional composition is a must for any contemporary Java developer these days.
Resources
Article Source Code:https://github.com/minborg/imperative-vs-declarative
SonarQube:https://www.sonarqube.org/
Speedment Stream:https://speedment.com/stream/
Speedment Initializer:https://www.speedment.com/initializer/
Published on Java Code Geeks with permission by Per Minborg, partner at our JCG program. See the original article here: Why Declarative Coding Makes You a Better Programmer Opinions expressed by Java Code Geeks contributors are their own. |
A big improvement! With this Java is starting to look almost as good as C# :)
c what?
Why do most Java programmers were glasses?
Most of them don’t C# :D
Very interesting article, but one thing to say:
The one that is using a double for money values has no clue of it all ;)
I know it is not part of the problem you address, but since here are a lot of beginners, please remove that
“Superior code metrics” is not the same as a “superior code”.
I don’t understand, all the “declarative” examples use the java stream API. So are the java stream API and declarative coding the same thing?
Not always. But the stream API is one mechanism to achieve a more declarative approach. If you take a look at most ‘functional’ languages you will see that most programs written in them are written in such a declarative manner. The Streams API provides many of the same tools so that you can do much the same thing in Java.